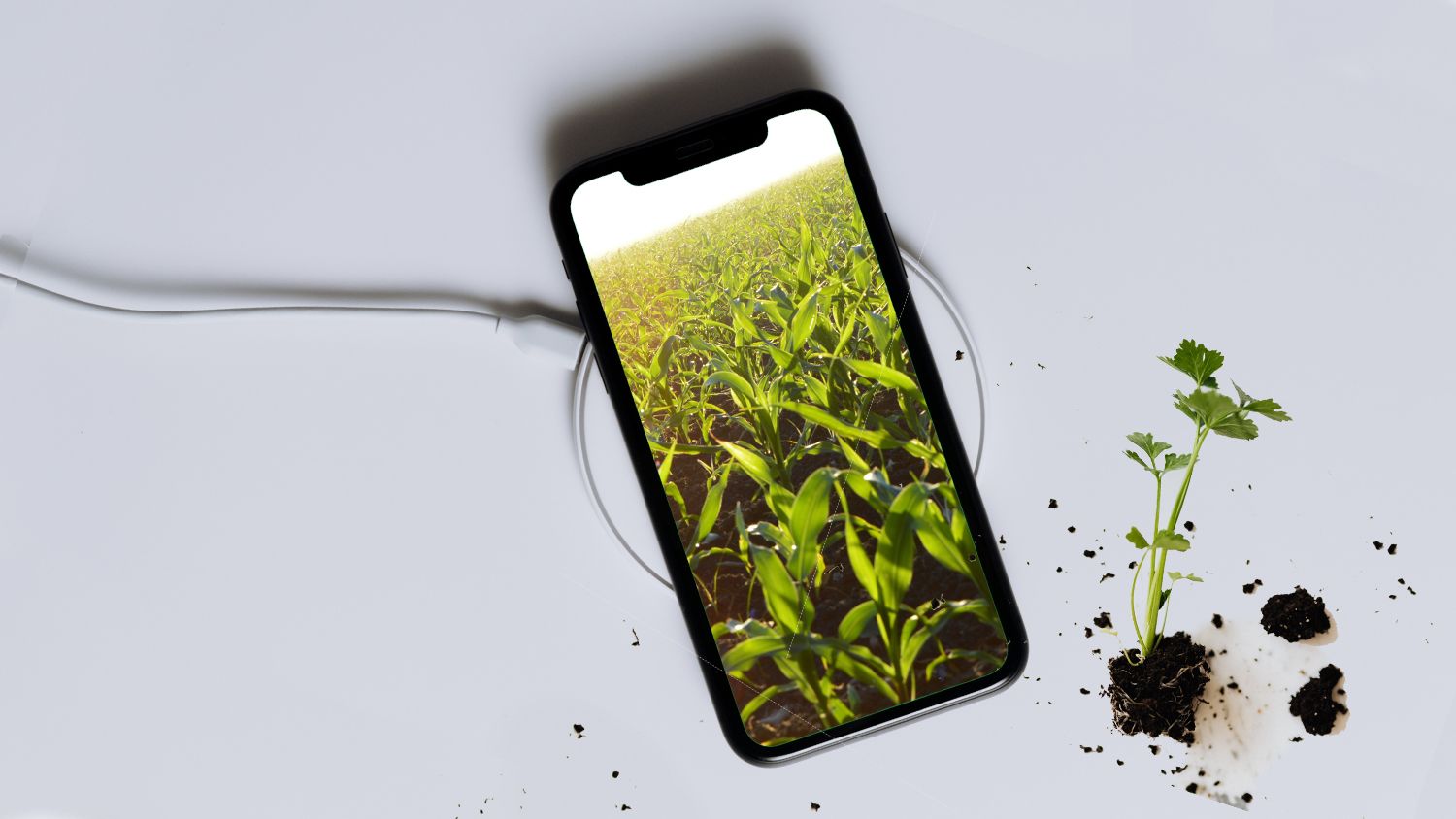
Cover crop use was steadily rising on farms in the early 2000s, with 60% of corn growers reporting their use. But in recent years consistency has wavered.
“Many growers see cover cropping as a more complicated system than traditional tillage. It may have soil benefits, but it was another layer of methodology that often interrupted seasonal workflow,” said Ramon Leon, weed science researcher and associate professor in the Department of Crop and Soil Sciences. “Now, the devastating economic impact of herbicide-resistant weeds is driving growers’ interest in alternative weed management. That’s where cover crops shine.”
The United States Department of Agriculture’s Natural Resources Conservation Service (NRCS) has awarded a three-year, $1.5M grant to NC State’s Department of Crop and Soil Sciences to develop a three-dimensional (3D) imaging system to visually map, treat, and predict herbicide-resistant weeds in cover crops.
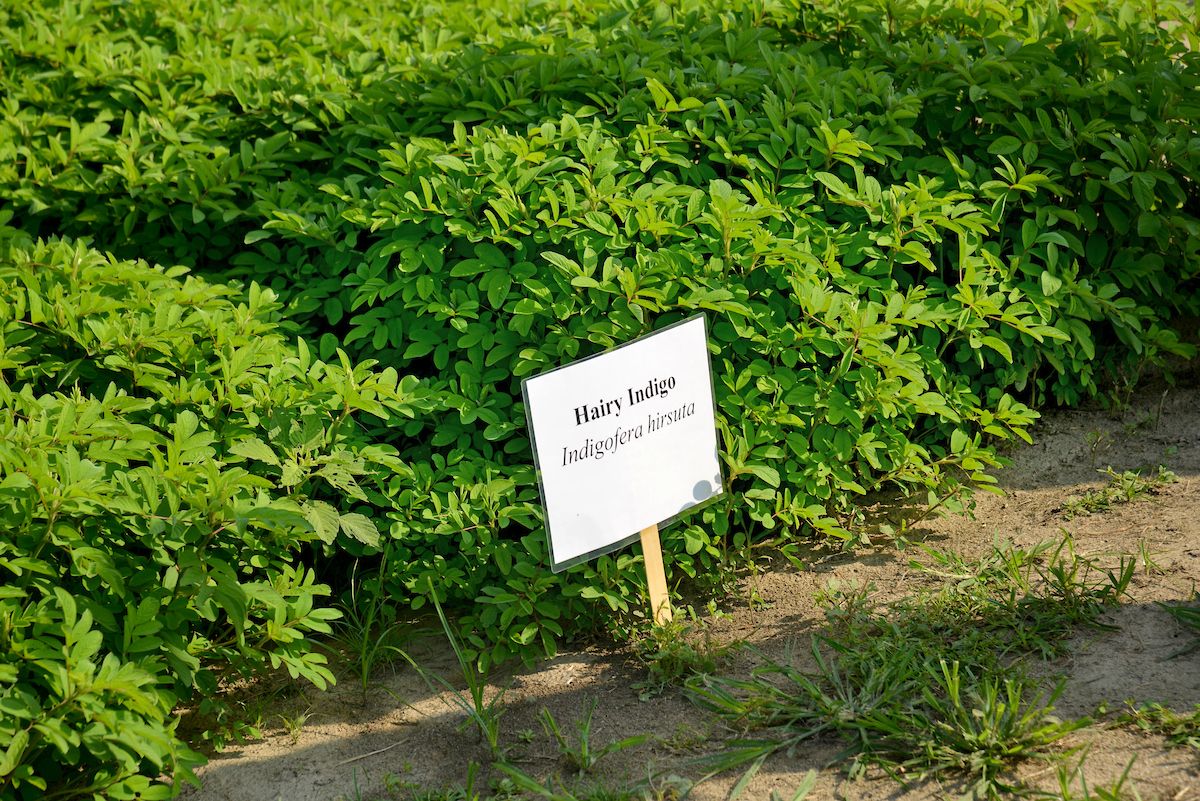
Alternative Weed Management
Cover crops gained favor among farmers and government agencies, primarily due to the crops’ agronomic and conservation value. These off-season plantings like rye, clover, and winter peas improve soil health through a multitude of benefits including increased soil organic matter, water retention, and nutrient levels all while reducing erosion and pests – including weed pressure. A seemingly win-win proposition.
The natural weed competition from cover crops reduces growers’ reliance on herbicide chemistry and soil disturbance for weed control. “But we’ve found that successful weed suppression by cover crops requires a robust stand. Otherwise, growers often revert to heavy tillage and chemical tank mixes to address multiple herbicide-resistant weeds,” Leon said.
3D Plant Vision System
Leon’s interdisciplinary 3D Weed Vision System aims to equip farmers with DIY imaging technology to reliably anticipate their cover crop’s performance and address weed escapes with precision accuracy. The interdisciplinary and multi-university team includes researchers from NC State, Texas A&M, Iowa State, and the University of Arizona.
Identifying Weeds in 3D
The three-phase project starts with a 3D computer vision system that uses real-time video from consumer-grade cameras (like GoPros) to render 3D imagery of field plants. The initial task is to identify weeds vs desirable plants and assess biomass. A cloud computing system will process data in the field and transmit a visual map to a mobile app.
“We developed an algorithm for 3D reconstruction using Structure from Motion (SfM),” Leon said. This open-sourced software platform uses multiple overlapping images from different angles to construct 3D high-resolution digital surface models. This methodology is often used for archaeological building reconstruction, geology, and tree biomass study. But the density and growth stages of plants increase the database complexity.
“SfM 3D-reconstruction is a simple technique using low-cost technology, which delivers impressive levels of detail. It has great potential for use in drone, tractor, or handheld phenotyping tool applications in agricultural environments,” said Paula Ramos Giraldo, a research scientist in computer vision and machine learning. “However, large-scale SfM is a difficult task, as it requires high computational time.”
The programming is built on the open-sourced OpenCV platform funded by Intel and Microsoft Azure. The NC State team was recently awarded for their work in an OpenCV developer challenge and continues to work with the group on coding for this and future applications.

Building a DIY System
The team will test and optimize hardware and software components for ground (tractor-mounted) and aerial (drone) systems. They hope to ultimately provide schematics for users to construct their own low-cost image collection systems to use with the cloud platform and app.
“Our goal is to develop a system that is accurate and functions elegantly. But it also has to be small, affordable, and simple,” Leon said.
Scaling Machine Learning In The Field
The next phase is to calibrate and scale the platform for commercial use. Ramos Giraldo is working on the 3D algorithm for large-scale point clouds and also is creating a mathematical model to reliably relate the biomass of cover crops and weeds.
“Our technological challenge is two-fold: first, to estimate weed coverage and density, and then, more importantly, to translate these 2D image projections into 3D large-scale point cloud reconstructions to estimate weed biomass. All of this will be compiled by deep-learning algorithms to detect the weed classes and calibrate the system for biomass estimation and mapping,” Ramos Giraldo said.
The tool is intended to do more than locate weeds; it is designed to identify weed species and compare weed height and volume to predict crop growth competition. Armed with these insights, users can make precise weed management decisions.
The team plans to refine and proof test the system across up to 60 farms in three distinct and agronomically important growing regions: North Carolina, Iowa, and Texas.
“We are fortunate to have access to tools and collaborators with an extensive on-farm network. This strength gives us access to a wide set of agricultural conditions to improve our models,” Leon said.
Teaching Drones to Intelligently Spot & Spray
Phase three will develop a protocol to target weed scapes with drone-based, site-specific management. Researchers will use the image vision system maps to identify and geocode weed escapes within a field. Machine learning algorithms will then generate optimal drone fly-routes to navigate the shortest distance to the identified weed patches. Finally, a drone sprayer system will precisely deliver herbicides to the target weeds.
“This system will allow growers to identify weak spots in a cover crop stand and allow them to proactively anticipate weed issues within the cover crop and even predict future outbreaks in the following cash crop. Growers can then choose how to manage those hot spots – with drone spraying or traditional management.” Leon said.
Tracking Weeds to Predict Yield
It’s important to know that we can predict, not just react to weed outbreaks
Leon envisions a future project phase with a feedback loop that transfers the knowledge base to end-users. “Once we have a working system we will refine it. But it can’t be just another technological novelty. We must train agents and farmers on how to use these tools and interpret their data to make agronomic decisions that boost both their crop performance and bottom line in a sustainable manner.”
Looking for More Innovation?
Crop and Soil Sciences’ research impacts farmers and students, from the cloud into the field. Follow how our innovations affect agriculture and environmental science by joining our weekly newsfeed.
If you are a student interested in weed science or precision agriculture, investigate our undergraduate and graduate degree programs. Then join us for a guided email tour of our department and university. Enhancing NC agriculture through technology innovation is part of how we are growing the future.