Faculty Focus: A Breed Apart
Computational biologist Amanda Hulse-Kemp discusses her recent award and groundbreaking methods for breeding research
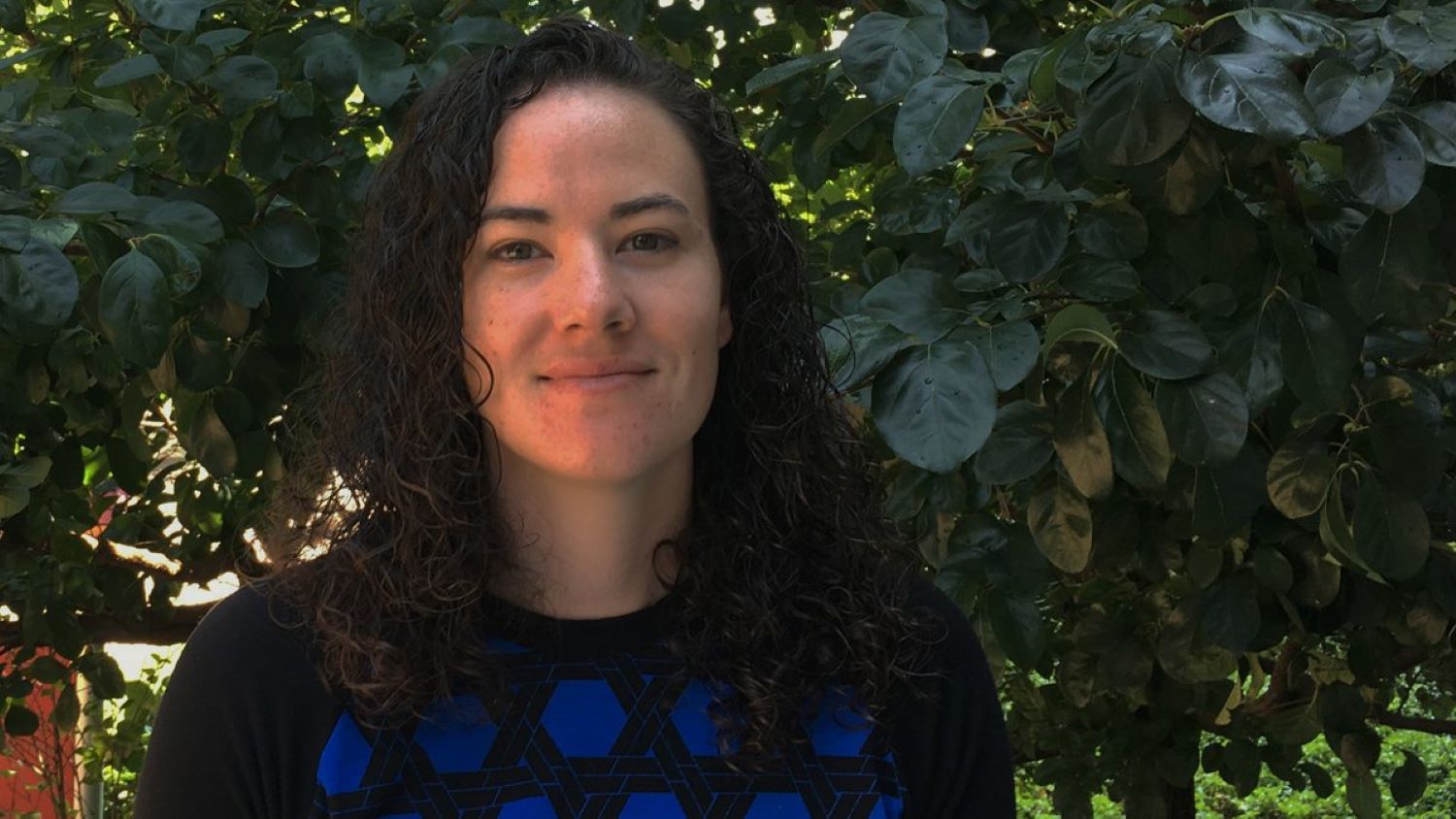
Amanda Hulse-Kemp crosses many fields, literally.
As a USDA assistant professor in computational biology at the College of Agriculture and Life Sciences, she helps NC State plant and animal scientists elevate their research by incorporating some of the latest computational advances, data-based research methods, and tools. In her main role as a scientist for the USDA Agricultural Research Service, she helps transition this basic research from the labs into the fields. She does this all while serving as an advisor for several Ph.D. students.
She has been involved in more than a dozen projects, including an initial research project with the N.C. Plant Sciences Initiative, which brings together scientists from a wide range of disciplines to solve some of the largest issues facing agriculture using big data, artificial intelligence, predictive modeling and other highly advanced methods and technologies.
Hulse-Kemp is also this year’s recipient of the Herbert L. Rothbart Early Career Research Scientist award, an honor given to only one USDA scientist per year, for leading projects that have rapidly advanced breeding programs for cotton, spinach, tomato, coffee, pepper, and other crops. Her work has allowed researchers to produce usable genetic data in three days, as opposed to weeks or months using earlier techniques.
Tell us about the research that led to your prestigious award.
I helped develop a process called high-throughput genotyping to support breeding programs and field decisions. This is about detecting a plant’s genetic traits to help determine which trait expressions will show up later in the field. For example, if we wait and observe the field we might find drought tolerance in one plant in the field. A few rows down, we may find another plant with a tolerance to fungal diseases. But now it’s about finding and cultivating these genetic and physical variations in plants much faster than traditional breeding programs – and using those findings to predict what the plant will do during the growing season. If a fungal disease shows up and you know the plant has the traits to tolerate it, a grower can avoid costly fungicide applications. We developed a method to collect data using a small credit card-like device that gives users 63,000 data points of genetic information for each plant. I used these techniques first on cotton crops, then developed similar research programs for spinach, tomato, coffee and pepper.
Why did you choose the field of Computational Biology?
I wanted to have an impact across many crops. Unlike breeders, who work usually on no more than a few commodities, computational biologists can work across a wide diversity of plant and animal species. As the genetics field advanced, researchers amassed giant datasets that required computational roles to handle them. That’s where I saw my opportunity to serve a wide range of plants—and animals. I still work on a few animal species. My philosophy is, “Genetics is genetics.” Whatever the crop or animal, our work advances farming and helps ensure that all people on our planet are fed – and clothed in the case of cotton.
Describe what it’s like to work at the intersection of genomics, biotechnology and crop breeding.
It’s super exciting. Farming is changing. We are rethinking how agriculture is done with the promise of exciting new technologies. Computational biology speeds up the research process. We were using a genotyping tool to select and screen plants in the field to find disease-resistant plants that might have bad traits associated with them, traits that lead to shorter size or lower yield. We were screening for those bad traits to ensure our new disease-resistant varieties had as few bad traits as possible as we advanced the breeding process. We were able to screen through 20,000 individual plants in two months and came up with a small handful of individual ones. Think about how much time, space, and money the traditional scheme would cost, where you would select and evaluate 20,000 plants, grow these plants in the field or greenhouse, and try to expose the bad traits through disease inoculation and other methods. It would add years to the research. That’s part of the push for using computational biology. It makes a faster, better end result for research that is essential to feeding all the people on this planet.
How do you see computational biology elevating the research at CALS?
Nearly all of the projects are impacted by computational biology, just at different levels. It allows us to elevate the research in ways we wouldn’t have been able to do without it. Take the new N.C. Plant Sciences Initiative, for example. We’re amassing big datasets that – coupled with certain technologies like artificial intelligence (AI) and machine learning – solve multiple problems in agriculture. I worked on a project with Jeffrey Dunne where we incorporated high-throughput genotyping for the detection of disease resistance in peanut fields. That same data can be used for other plant disease studies. As we collect this data, we can then move to the “what now” phase, where we figure out all the ways we can use this information. It’s about how you keep, set up, and store the data to create new projects. It’s super powerful.
It’s a great partnership between NC State and USDA-ARS. We start off with basic research at NC State and readily move on to the applied research through ARS. I get to see much of the work translate to the field through this role, which is very rewarding.
What do you enjoy most about your faculty appointment at NC State?
Mentoring. I love having the ability to help build the next generation of researchers who will probably be much smarter than I am. That’s what I tell students. I strive for them to be smarter than me when they’ve finished their graduate program, then I’ve done a good job.
Why is computational biology so important?
Computational biology lets you answer a lot of different questions with the same datasets, which can be very powerful. You can have two researchers working on the same datasets but answering entirely different research questions and projects. This is not really possible using other approaches.