Plant Breeding AI Gets Schooled in New Student Competition
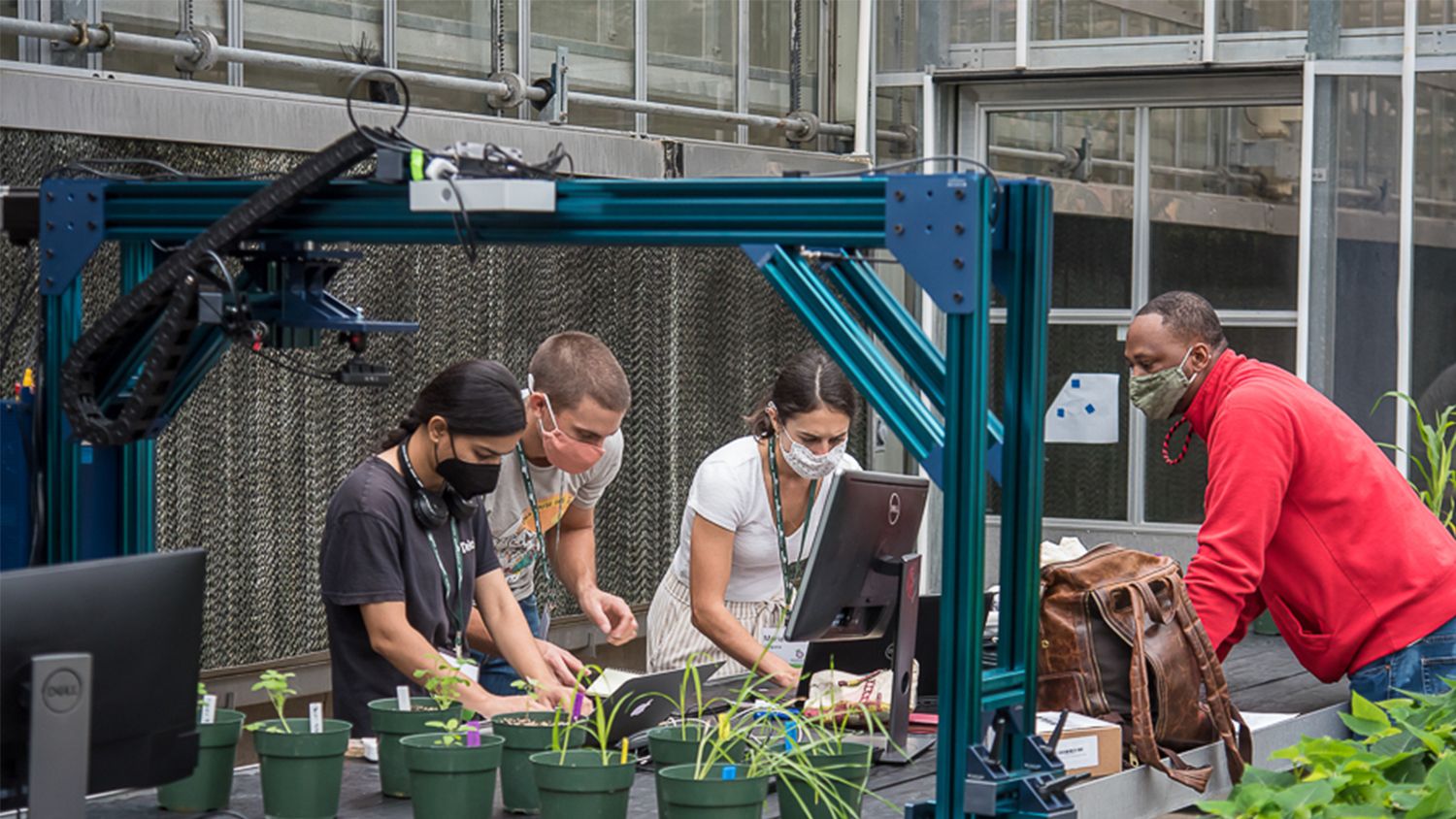
If Hillsborough Street glowed a bit brighter recently, it might have been from the collective mental energy focused on NC State’s first Agricultural Technology Hackathon. The university and USDA-Agricultural Research Service created the Ag Tech Hackathon to accelerate agricultural research using computer vision, machine learning, and robotics.
Phenotyping is time-consuming and labor-intensive for researchers. NC State and the USDA-ARS jointly developed a low-cost, open-source solution for non-destructive high-throughput phenotyping in greenhouses, affectionately called the BenchBot. The team recently won third place regionally in the OpenCV Spatial AI Competition with the BenchBot and decided to host a similar competition for students.
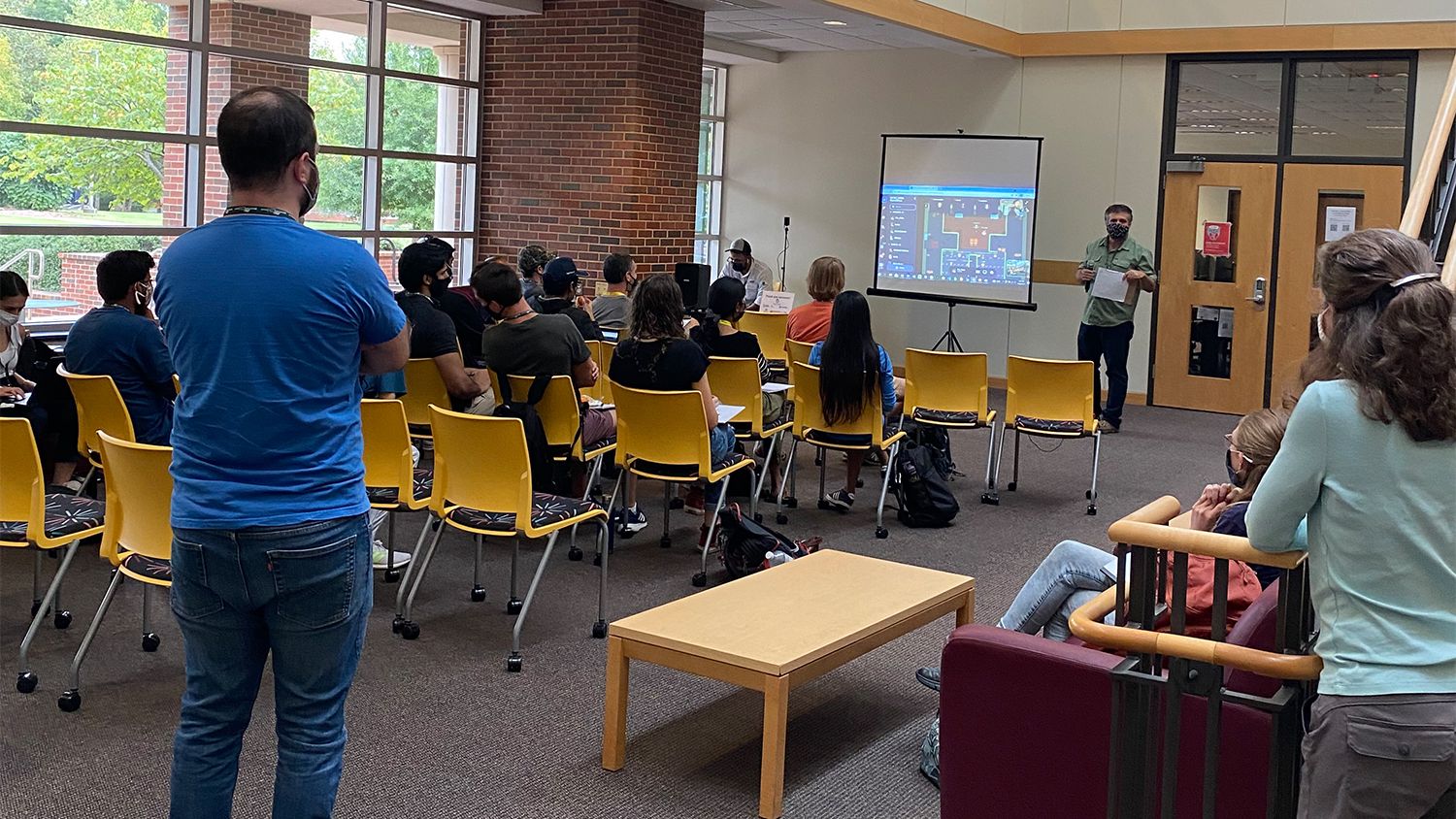
Chris Reberg-Horton, an NC State Department of Crop and Soil Sciences professor and the Resilient Agricultural System Platform Director in the university’s Plant Sciences Initiative, helped conceptualize the student competition event.
“The idea of a hackathon is to drive innovation,” Reberg-Horton said. “But innovation is not just about creating new things; it’s about connecting functions, techniques or technologies to solve a real problem. When we think of computer science and engineering applied to agriculture, we need more enthusiasts who are motivated to participate in the big challenges in the field: detecting pests and other plant stressors, interpreting data from smart farm equipment and sensor networks and increasing the autonomy of farm equipment. These solutions necessitate new thinking, which is why students are an excellent complement to this technological development.”
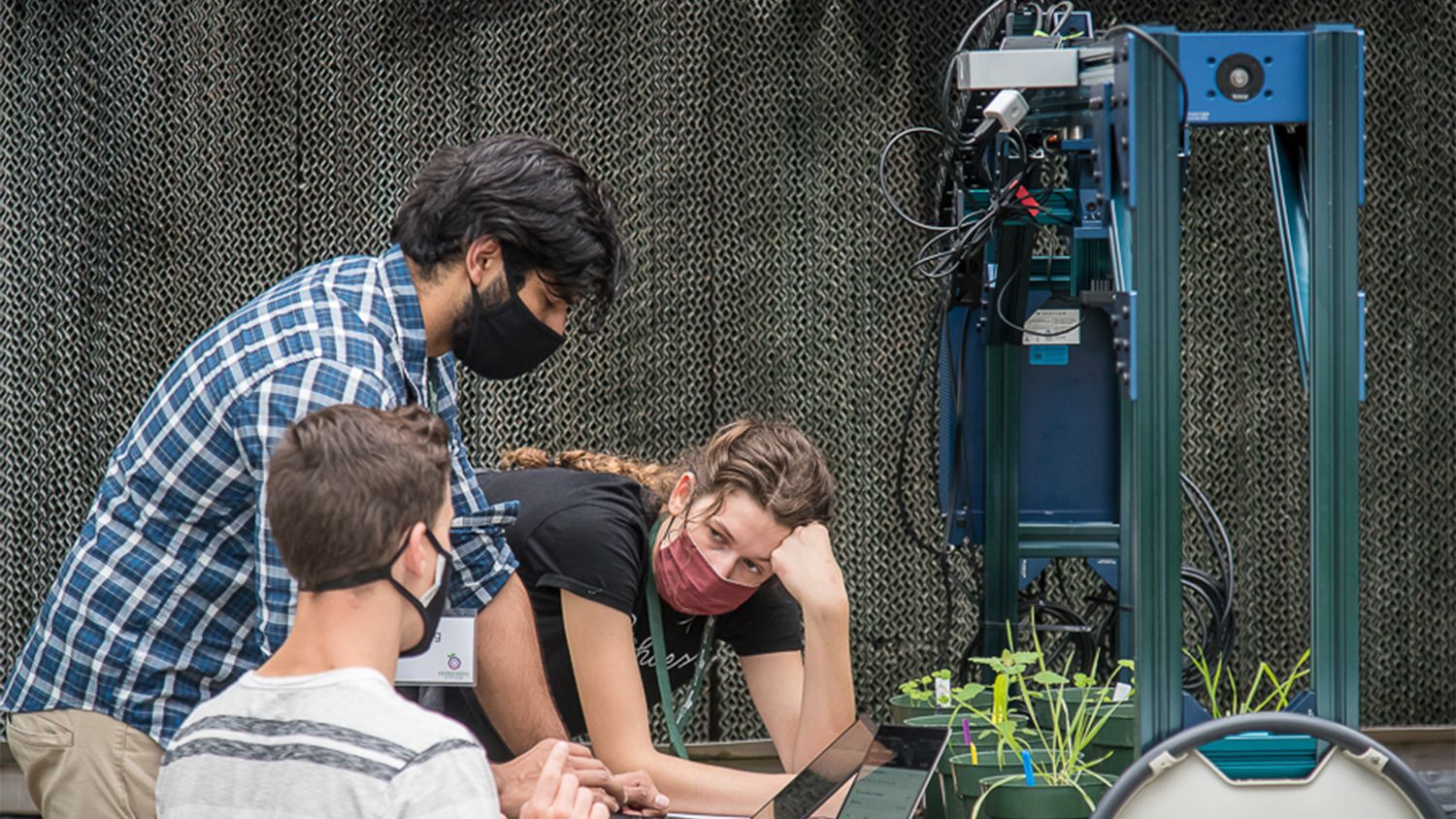
Hackathon Categories and Winners
Hackathon participants competed in three categories, all using the BenchBot to sense and interpret greenhouse plant growth.
- Hardware
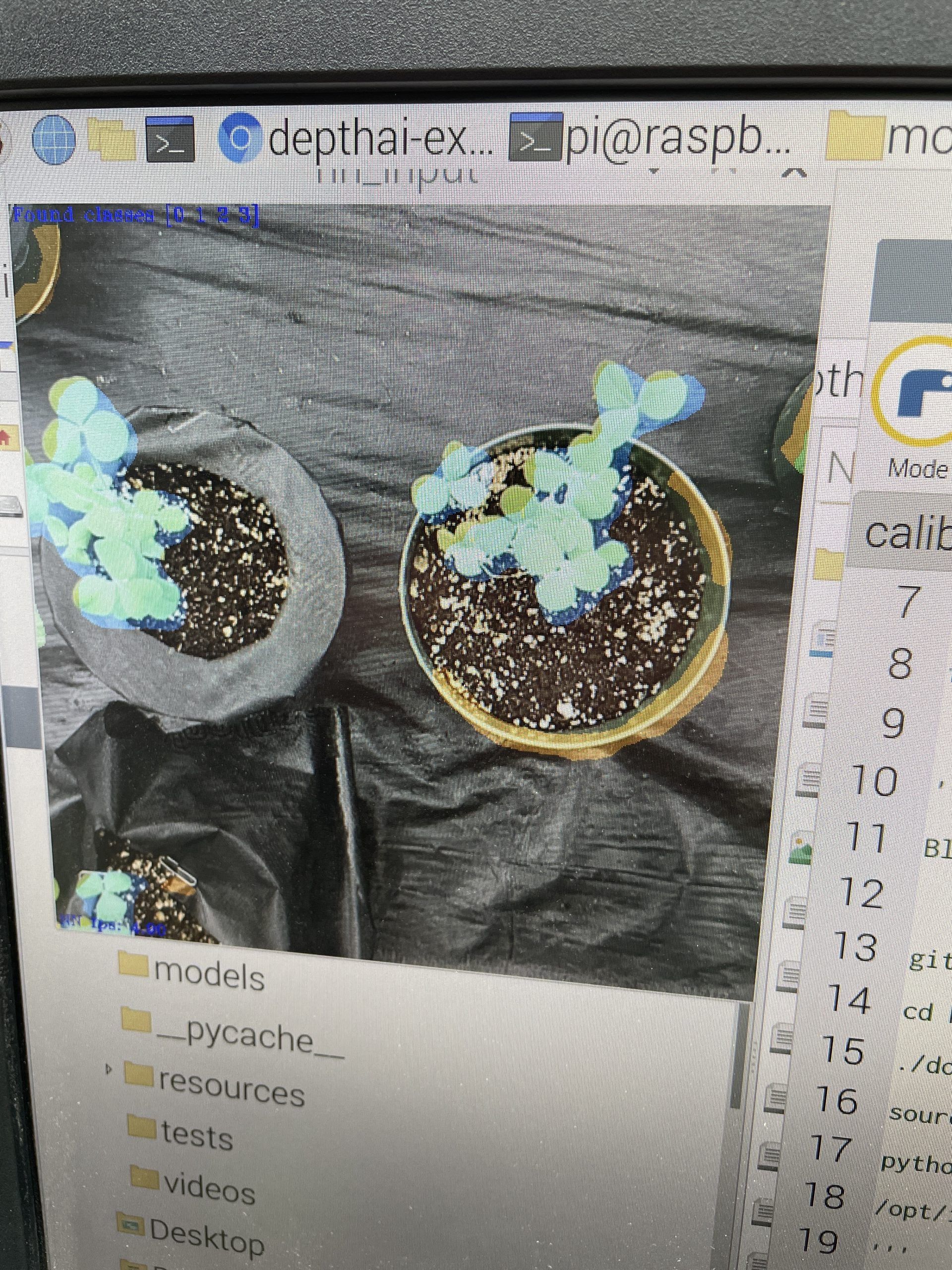
Specifically designed for electrical and computer engineers, this category required participants to pair a single board computer (like Raspberry Pi) with an artificial intelligence camera (RGB+depth) to create a novel, scalable solution for digital agriculture.
Hardware category winners: Dewang Tara, Caleb Wheeler and Cassidy Petrykowski; NC State Department of Electrical & Computer Engineering
- Deep Learning
Budding data scientists applied their machine learning and computer vision skills to real use cases in agriculture. Participants were given a large annotated agricultural image dataset to achieve accurate prediction models.
Deep Learning category winners: Anjali Garg, NC State Department of Computer Science; David Peery, UNC Department of Computer Science; and Chinmay Savadikar, NC State Department of Electrical and Computer Engineering
- Roboflow
Organizers created this category for biology and agriculture students without a background in computer science or electrical engineering. Participants were introduced to Roboflow’s drag-and-drop system for machine learning and were then challenged to load the trained algorithm into a web application.
Roboflow category winners: Susmita Gaire and Greta Rockstad; NC State Department of Crop and Soil Sciences
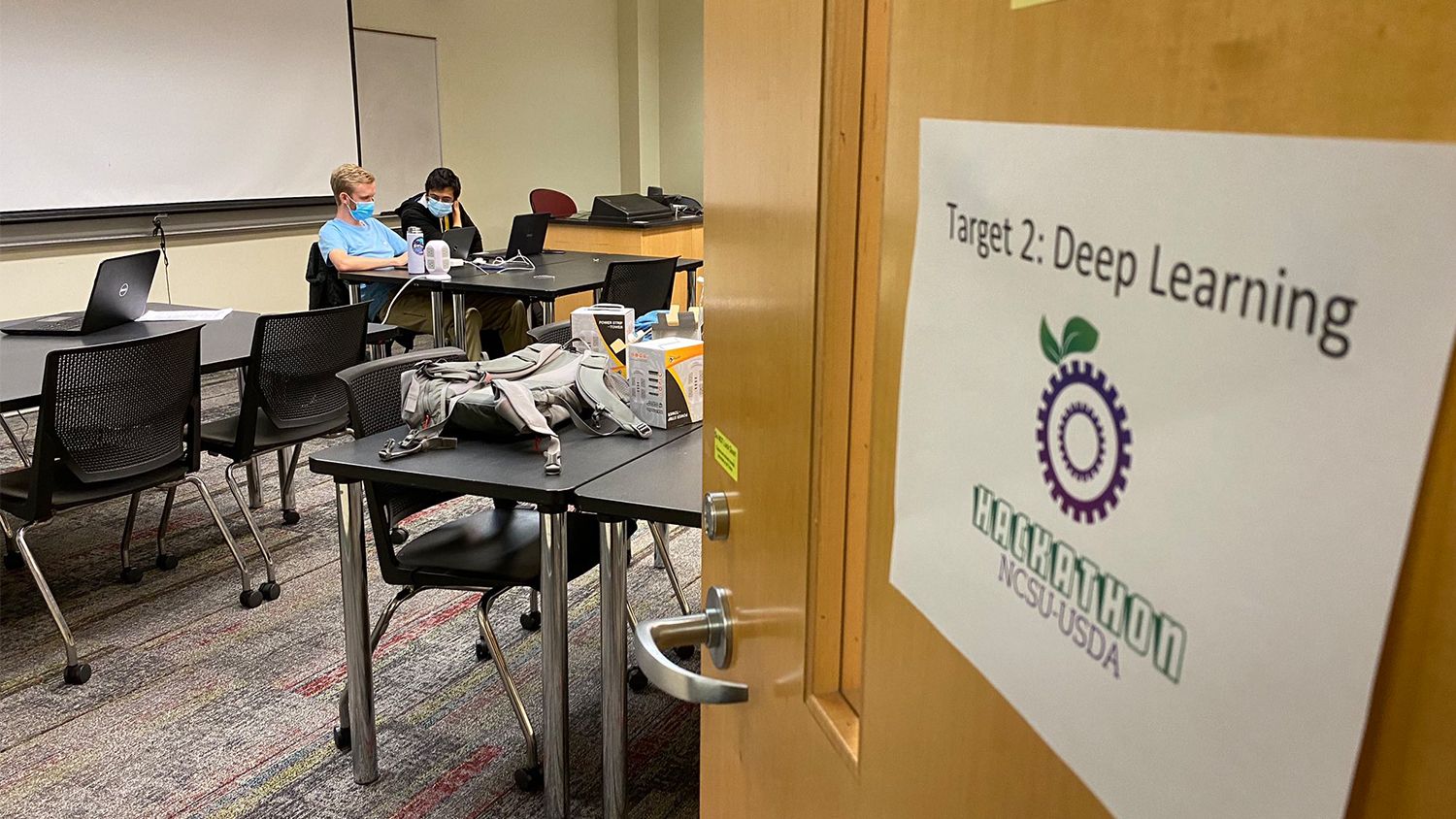
Resiliency Rewarded
Greta Rockstad is an NC State turfgrass master’s student. After some trial and error, she and her turfgrass teammate Susmita Gaire hit their stride using research data from a fellow student.
“We ended up using images of a large patch trial in zoysiagrass collected by another graduate student in our lab, Kirtus Houting. While not collected with the intention to be used in a machine learning model, his dataset was ideal because his disease ratings were distinct classes, and classification was a model that Roboflow could fit,” Rockstad said.
The team recognized that their model’s accuracy was too low for immediate practical use but realized that the experience was their true prize.
“I think in our presentation we demonstrated that we had several challenges and had to change our strategy to overcome them, and that was a compelling narrative for the judges because considering that our objective, if any, was to learn, we most definitely did that.”
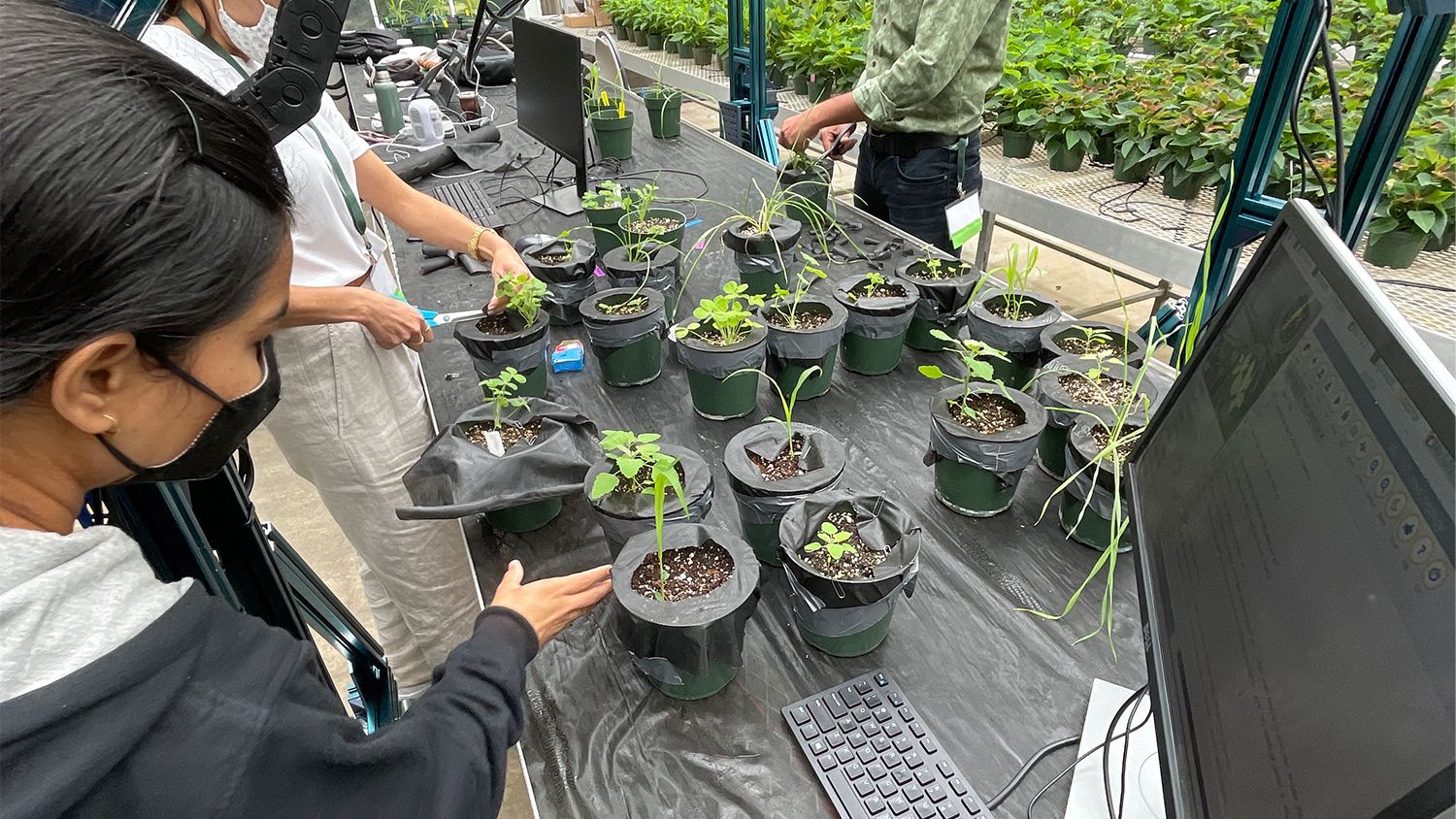
An Ag Technology Marathon
A total of eight teams competed in the two-day event for cash prizes and bragging rights. Teams participated virtually via gather.town and in-person at NC State’s Fox Laboratories. Organizers constructed three in-greenhouse BenchBots on which participants could create and test their deployments. The event format’s flexibility allowed hackers to listen to the event virtually yet work locally with their team.
“In the Hackathon, we were looking for participants to join a technology marathon with different challenges,” said Paula Ramos-Giraldo, BenchBot creator and former crop and soil science researcher. “We wanted to simultaneously expedite current research questions and allow students to learn, build and share their creations during two days of competition.”
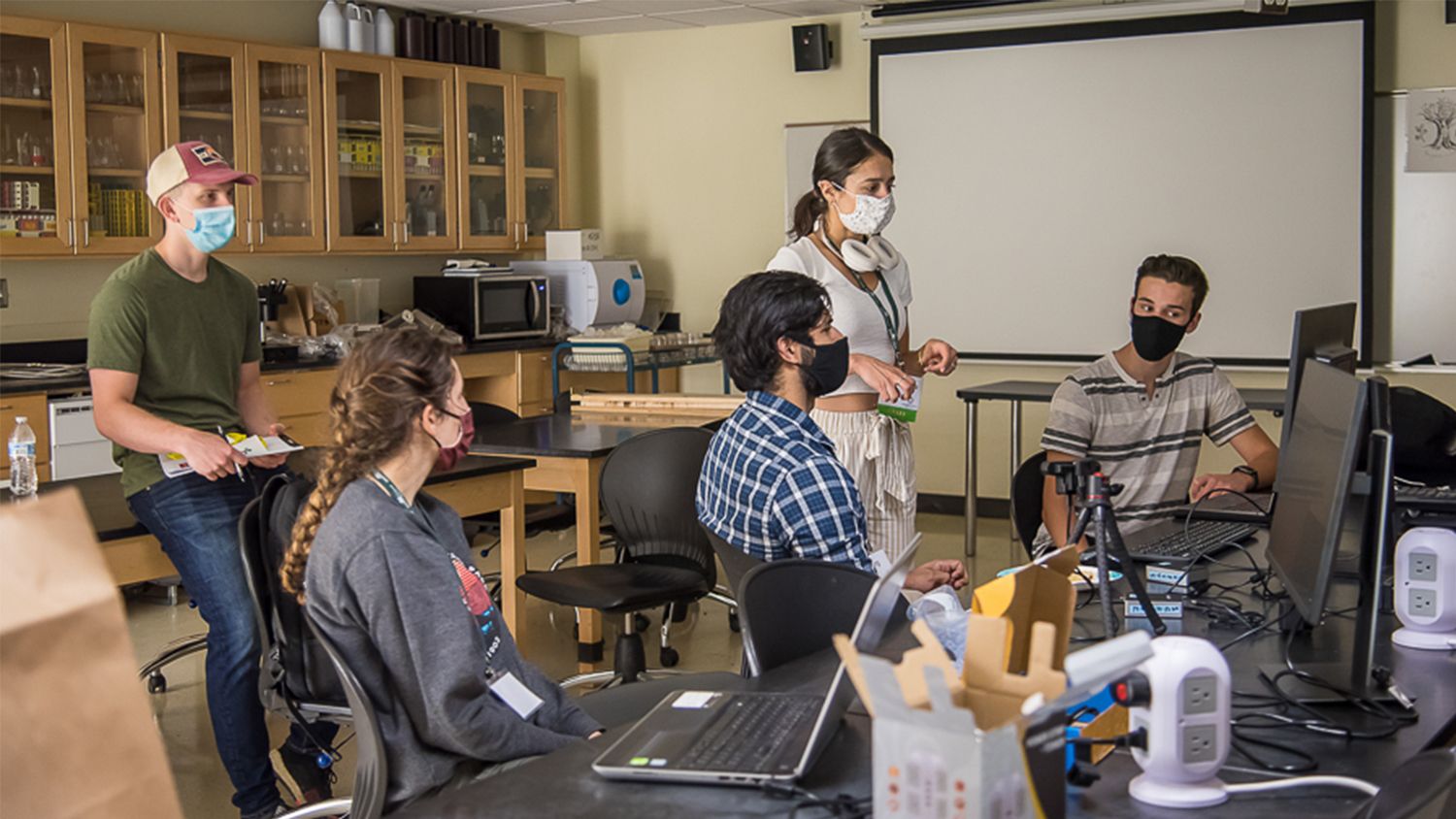
The event was sponsored by OpenCV, Luxonis, Microsoft, and SAS of Cary, which provided funds for the competition awards.
Steven Mirsky, a research scientist with USDA-ARS’s Sustainable Agricultural Systems Laboratory, co-hosted the event with NC State.
“Bringing together engineers with applied scientists to innovate on real-world solutions is critical to building productive, efficient, and resilient food systems. It’s really exciting to see NC State and USDA leverage their respective strengths in fostering this culture of innovation with the next generation of scientists.”
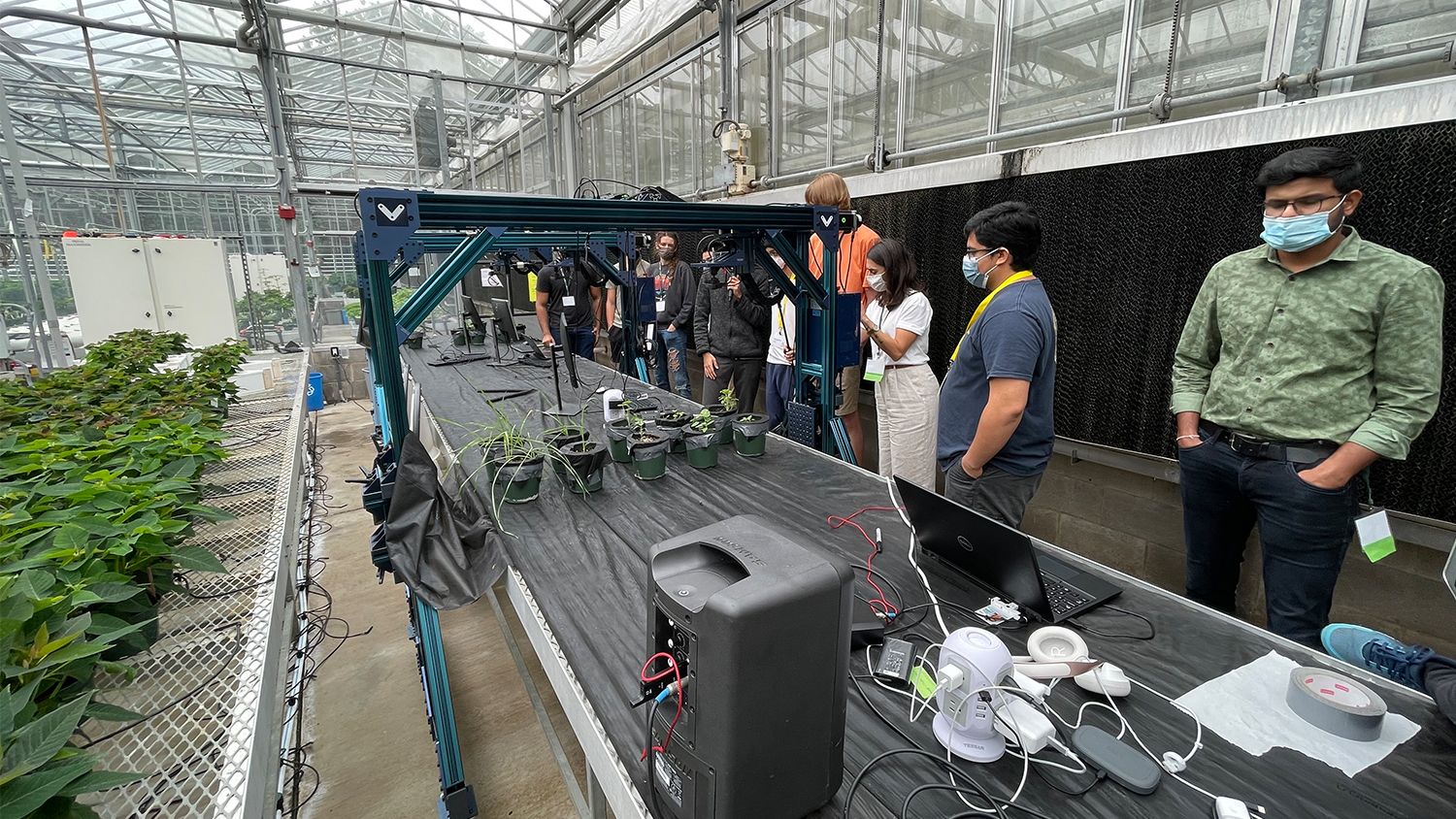
Training To Leave The Greenhouse
BenchBot is a plant phenotyping platform consisting of two main components: an RGB+depth camera and a processing unit to control the platform and camera movement.
Researchers have successfully used the BenchBot’s greenhouse-acquired images to train machine learning algorithms under controlled conditions and are now refining the algorithms to detect and identify plants, detect leaves and determine leaf area, and estimate total plant biomass for field use.
Three BenchBot robots will be housed in the new Plant Sciences Building, a new $160 million project at NC State that will foster interdisciplinary research on agriculture’s grand challenges. One BenchBot also resides at the USDA-ARS Beltsville, Maryland facility.
- Categories: