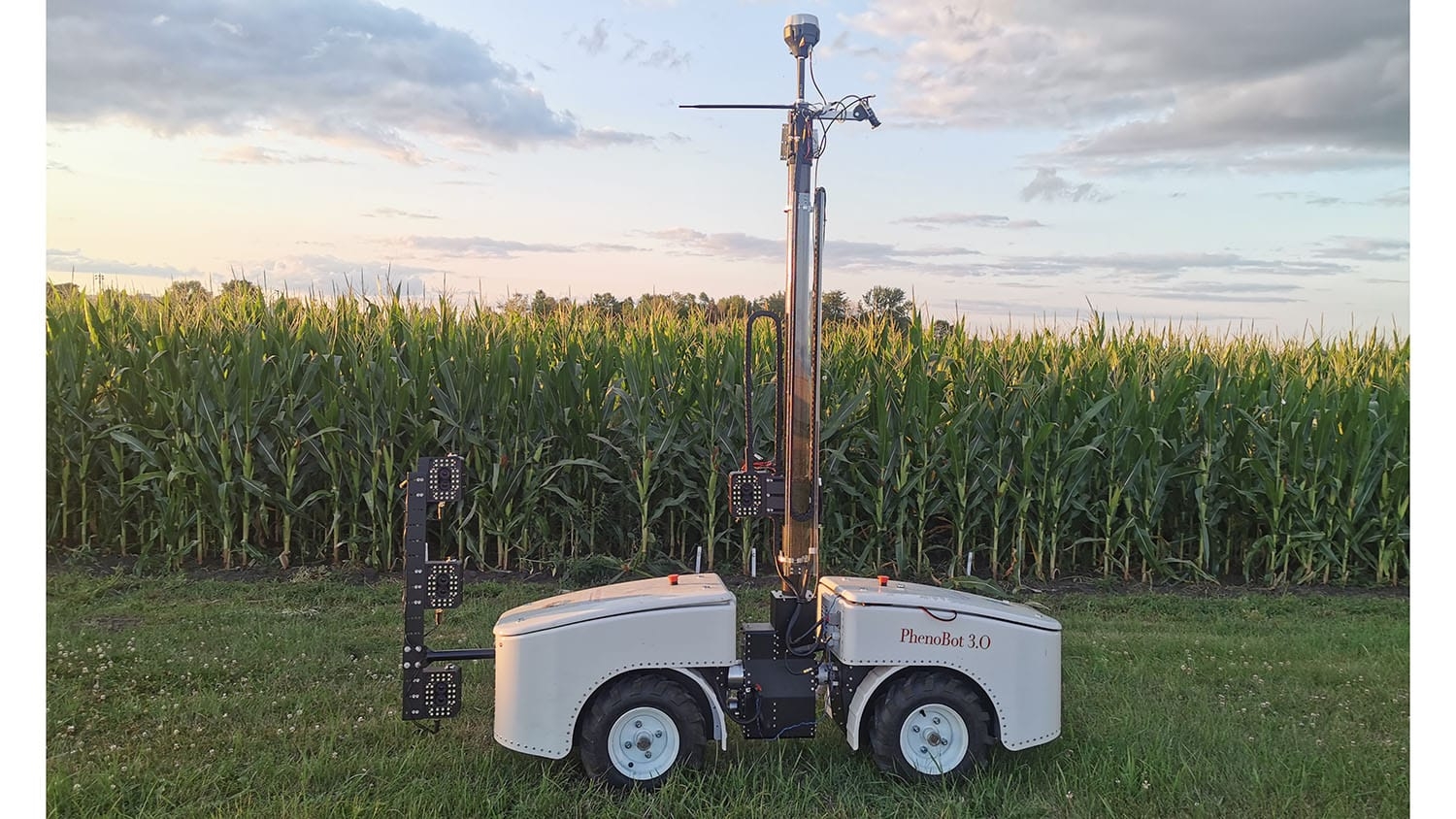
Researchers from North Carolina State University and Iowa State University have demonstrated an automated technology capable of accurately measuring the angle of leaves on corn plants in the field. This technology makes data collection on leaf angles significantly more efficient than conventional techniques, providing plant breeders with useful data more quickly.
“The angle of a plant’s leaves, relative to its stem, is important because the leaf angle affects how efficient the plant is at performing photosynthesis,” says Lirong Xiang, first author of a paper on the work and an assistant professor of biological and agricultural engineering at NC State. “For example, in corn, you want leaves at the top that are relatively vertical, but leaves further down the stalk that are more horizontal. This allows the plant to harvest more sunlight. Researchers who focus on plant breeding monitor this sort of plant architecture, because it informs their work.
“However, conventional methods for measuring leaf angles involve measuring leaves by hand with a protractor – which is both time-consuming and labor-intensive,” Xiang says. “We wanted to find a way to automate this process – and we did.”
The new technology – called AngleNet – has two key components: the hardware and the software.
The hardware, in this case, is a robotic device that is mounted on wheels. The device is steered manually, and is narrow enough to navigate between crop rows that are spaced 30 inches apart –the standard width used by farmers. The device itself consists of four tiers of cameras, each of which is set to a different height to capture a different level of leaves on the surrounding plants. Each tier includes two cameras, allowing it to capture a stereoscopic view of the leaves and enable 3D modeling of plants.
As the device is steered down a row of plants, it is programmed to capture multiple stereoscopic images, at multiple heights, of every plant that it passes.
All of this visual data is fed into a software program that then computes the leaf angle for the leaves of each plant at different heights.
“For plant breeders, it’s important to know not only what the leaf angle is, but how far those leaves are above the ground,” Xiang says. “This gives them the information they need to assess the leaf angle distribution for each row of plants. This, in turn, can help them identify genetic lines that have desirable traits – or undesirable traits.”
To test the accuracy of AngleNet, the researchers compared leaf angle measurements done by the robot in a corn field to leaf angle measurements made by hand using conventional techniques.
“We found that the angles measured by AngleNet were within 5 degrees of the angles measured by hand, which is well within the accepted margin of error for purposes of plant breeding,” Xiang says.
“We’re already working with some crop scientists to make use of this technology, and we’re optimistic that more researchers will be interested in adopting the technology to inform their work. Ultimately, our goal is to help expedite plant breeding research that will improve crop yield.”
The paper, “Field-based robotic leaf angle detection and characterization of maize plants using stereo vision and deep convolutional neural networks,” is published open access in the Journal of Field Robotics. Corresponding author of the paper is Lie Tang, a professor of agricultural and biosystems engineering at Iowa State. The paper was co-authored by Jingyao Gai, of Iowa State and Guanxi University; Yin Bao, of Iowa State and Auburn University; and Jianming Yu and Patrick Schnable, of Iowa State. The work was done with support from the National Science Foundation, under grant number 1625364; and from the Plant Sciences Institute at Iowa State.
-shipman-
Note to Editors: The study abstract follows.
“Field-based robotic leaf angle detection and characterization of maize plants using stereo vision and deep convolutional neural networks”
Authors: Lirong Xiang, North Carolina State University and Iowa State University; Jingyao Gaia, Iowa State University and Guanxi University; Yin Bao, Iowa State University and Auburn University; Jianming Yue, Patrick S. Schnablee and Lie Tang, Iowa State University
Published: Feb. 27, Journal of Field Robotics
DOI: 10.1002/rob.22166
Abstract: Maize (Zea mays L.) is one of the three major cereal crops in the world. Leaf angle is an important architectural trait of crops due to its substantial role in light interception by the canopy and hence photosynthetic efficiency. Traditionally, leaf angle has been measured using a protractor, a process that is both slow and laborious. Efficiently measuring leaf angle under field conditions via imaging is challenging due to leaf density in the canopy and the resulting occlusions. However, advances in imaging technologies and machine learning have provided new tools for image acquisition and analysis that could be used to characterize leaf angle using three-dimensional (3D) models of field-grown plants. In this study, PhenoBot 3.0, a robotic vehicle designed to traverse between pairs of agronomically spaced rows of crops, was equipped with multiple tiers of PhenoStereo cameras to capture side-view images of maize plants in the field. PhenoStereo is a customized stereo camera module with integrated strobe lighting for high-speed stereoscopic image acquisition under variable outdoor lighting conditions. An automated image processing pipeline (AngleNet) was developed to measure leaf angles of nonoccluded leaves. In this pipeline, a novel representation form of leaf angle as a triplet of keypoints was proposed. The pipeline employs convolutional neural networks to detect each leaf angle in two-dimensional images and 3D modeling approaches to extract quantitative data from reconstructed models. Satisfactory accuracies in terms of correlation coefficient (r) and mean absolute error (MAE) were achieved for leaf angle (r>0.87, MAE<5°) and internode heights (r>0.99, MAE<3.5cm). Our study demonstrates the feasibility of using stereo vision to investigate the distribution of leaf angles in maize under field conditions. The proposed system is an efficient alternative to traditional leaf angle phenotyping and thus could accelerate breeding for improved plant architecture.
This post was originally published in NC State News.